Following our live Progress Update event in May, we’re returning to our regularly scheduled programming here at the NewLimit Blog. Check out the video of our event if you haven’t seen it yet.
Through the spring and early summer, we’ve focused on developing functional assays for Immunology & Metabolism programs, improving the efficiency of our Discovery Engine screens, and revving the Engine to construct our reprogramming data corpus.
A few highlights by the numbers, quarter-over-quarter:
2X reduction in the cost per transcription factor (TF) set tested
1.7X the number of TF sets tested
1.5X the number of TF sets that reverse the phenotypic age of T cells
+4 functional assays for hepatocyte cell age
+1 functional assay for T cell age
+3 team members
+1 SAB member
People
We’ve also been excited to welcome new members to our team:
Yu-Hsuan Lin joined as a Scientist II on our Metabolism team. Yu-Hsuan previously trained with our SAB member Hao Zhu and developed CAR-NK therapies at Nkarta.
James Briggs joined as a Senior Scientist on our Write (Epigenetic Editing) team. James previously trained with Allon Klein and Marc Kirschner at Harvard and Feng Zheng at the Broad.
Fatima Rizvi joined as a Scientist on our Metabolism team. Fatima previously trained with Valerie Gouon-Evans at Boston University.
Improving the efficiency of our Discovery Engine
Our Discovery Engine is designed to test partial reprogramming interventions at scale. Our goal is to explore as many hypotheses as possible on a given budget. If we can reduce the cost of testing each hypothesis, we can test more of them, increasing our chances of finding reprogramming factors that reverse cell age.
Over the past few months, our Write (Epigenetic Editing) and Read (Single Cell Genomics) teams developed two new approaches to increase the number of interventions we can test per dollar. First, our Write team developed a strategy to reprogram and purify cells more efficiently in our screens, decreasing the number of cells we need to profile to test each set of factors by 1.4X. Our Read team then developed a new single cell genomics chemistry that decreases the cost of each cell profile 1.4X.
In our most efficient Engine experiments, these changes combined reduced the cost per TF set tested by 2X.
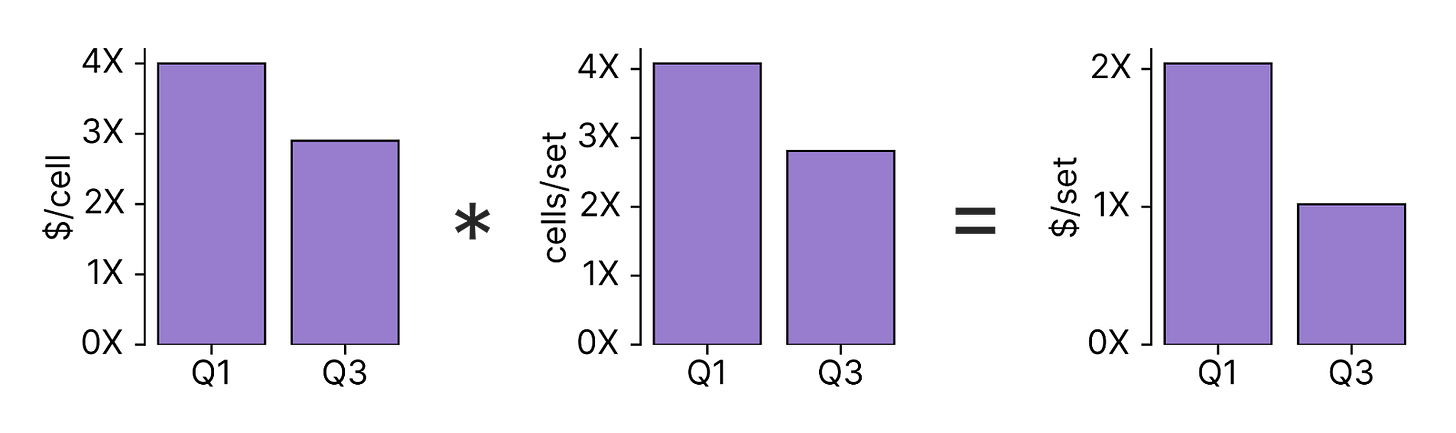
Reversing phenotypic age in primary human T cells
Our Discovery Engine screens are only as impactful as the biology they reveal. The primary question we ask in each of our screens is whether we’ve made an old cell look young based on its genomic profile. Concretely, we use machine learning models that predict cell age from cell genomics profiles to measure whether a set of transcription factors (TFs) can shift an old cell to a younger looking state.
Over the past few months, we 1.5X’d the number of TF sets we’ve discovered that can reverse phenotypic age in primary human T cells. Among these hits, we’ve found that many TFs outside the Yamanaka Factor set can shift phenotypic age. Cell age reprogramming appears to be broader than just the first factors that the field discovered.
We also found that many of these TF sets can reprogram cell age durably, even after the TFs themselves have been turned off. There are still many questions to be answered, but these early results suggest that partial reprogramming for a brief time may lead to lasting changes in cell age. Check out our Progress Update video for a deep dive on a subset of these results.
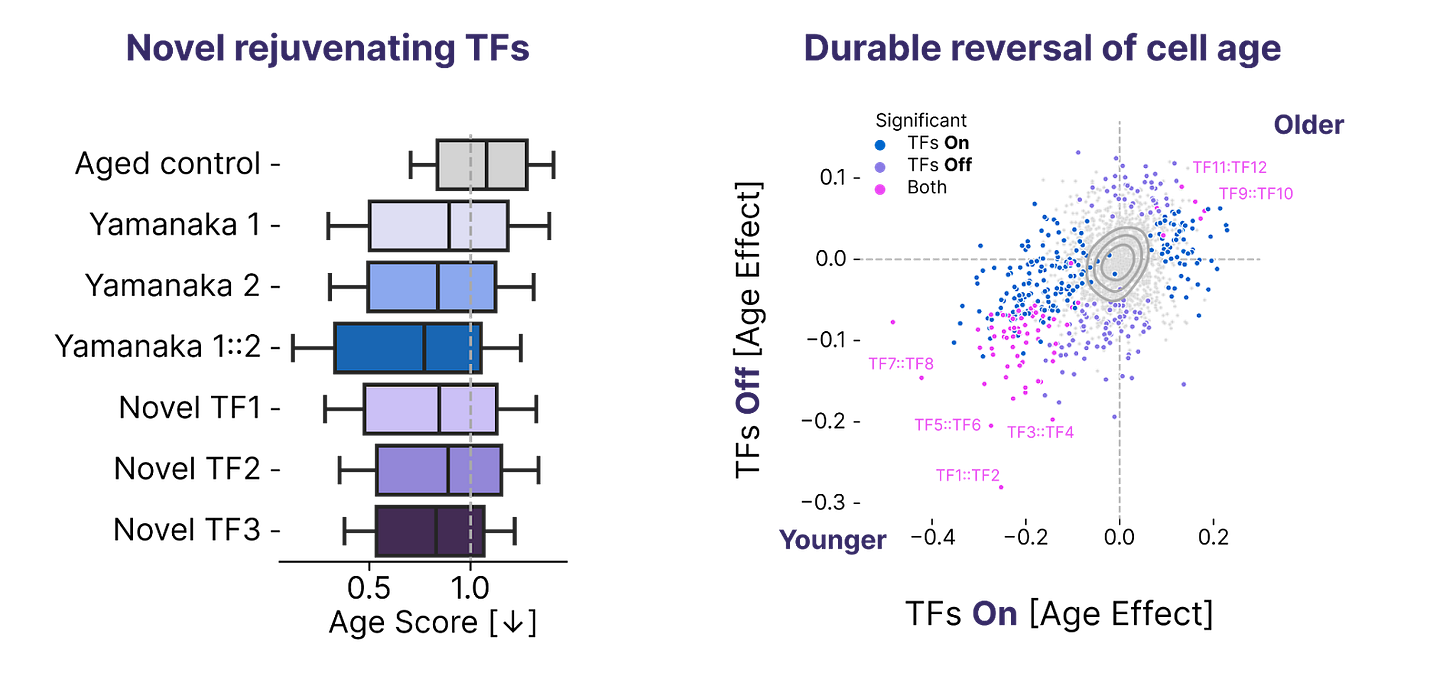
Functional assays for T cell age
While reversing phenotypic age is exciting, reversing functional age is our ultimate goal. We want our reprogramming interventions to make old cells act young, not just look young.
Each of our Therapeutic teams are developing assays to measure the functional impact of reprogramming therapies on cell age. The first step in this journey is developing assays that can discriminate between young and old cells in the first place.
We previously reported on a set of functional assays that can discriminate between young and old human T cells. We’re excited to share some early data from one of these assays alongside our live Progress Update.
T cells are distributed across a range of distinct functional states, each contributing to different features of the immune system. Our team discovered that young and old T cells adopt different functional states in one of our assays, and this distribution of states is sufficient to reliably determine if a tube of T cells came from a young or old person.
That final point is worth emphasizing – the function of young and old T cells is distinct enough that you can reliably predict a person’s age just by measuring how their T cells perform in the laboratory.
Over the past two months, we’ve found another functional assay for T cell age to add to our repertoire and look forward to sharing results in the future.
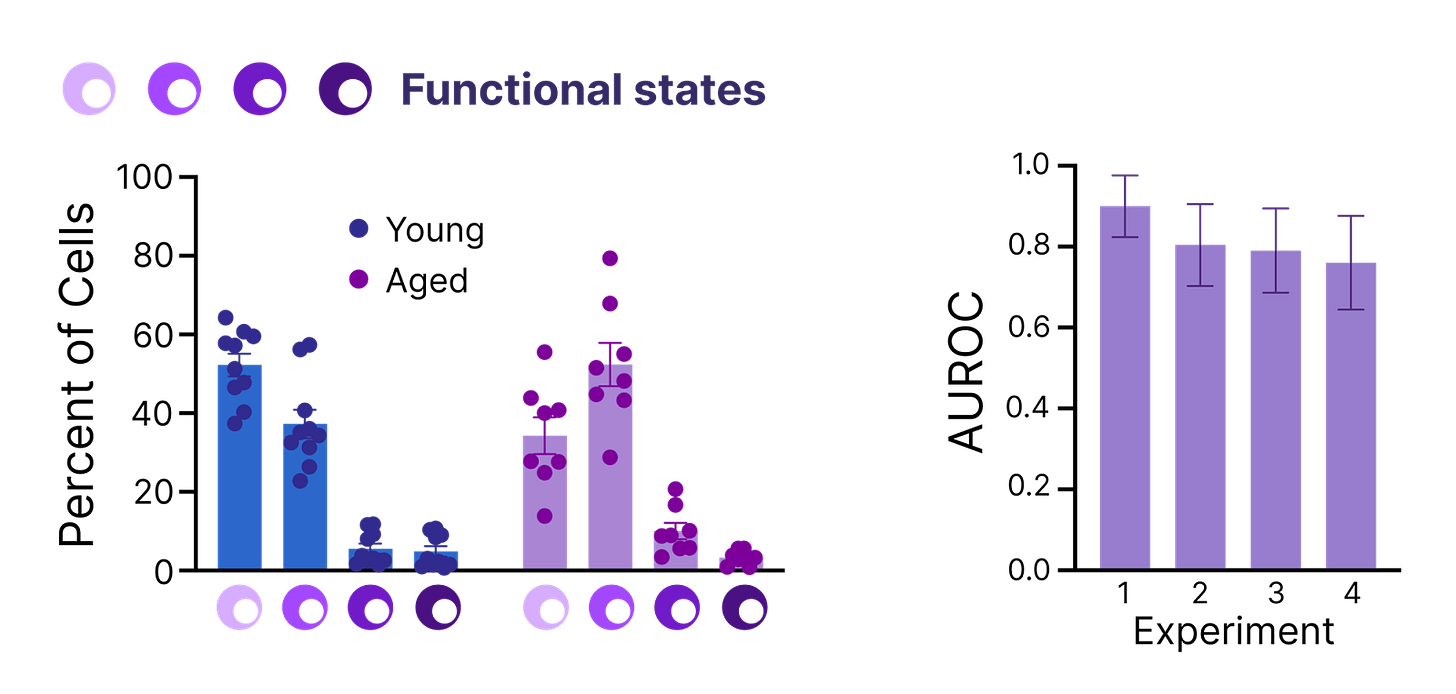
Functional assays for hepatocyte age
In addition to T cells, we’re developing reprogramming medicines to reverse cell age in hepatocytes. We’ve been building our Metabolism program throughout the spring, and we’re excited to share that our team has already developed our first set of functional assays for mouse and human hepatocyte age.
We found that young hepatocytes are significantly more regenerative than old cells, and this increased regenerative potential can protect young animals from insults that are debilitating for old animals. We also found metabolic changes that are sufficient to discriminate young and old hepatocytes in humans. Similar to our T cell assays, these differences are sufficient to predict the age of a human donor based only on how their hepatocytes perform in the laboratory.
These functional assays form a critical component in our Discovery Engine and their development represents a meaningful milestone for our newest Therapeutic team.
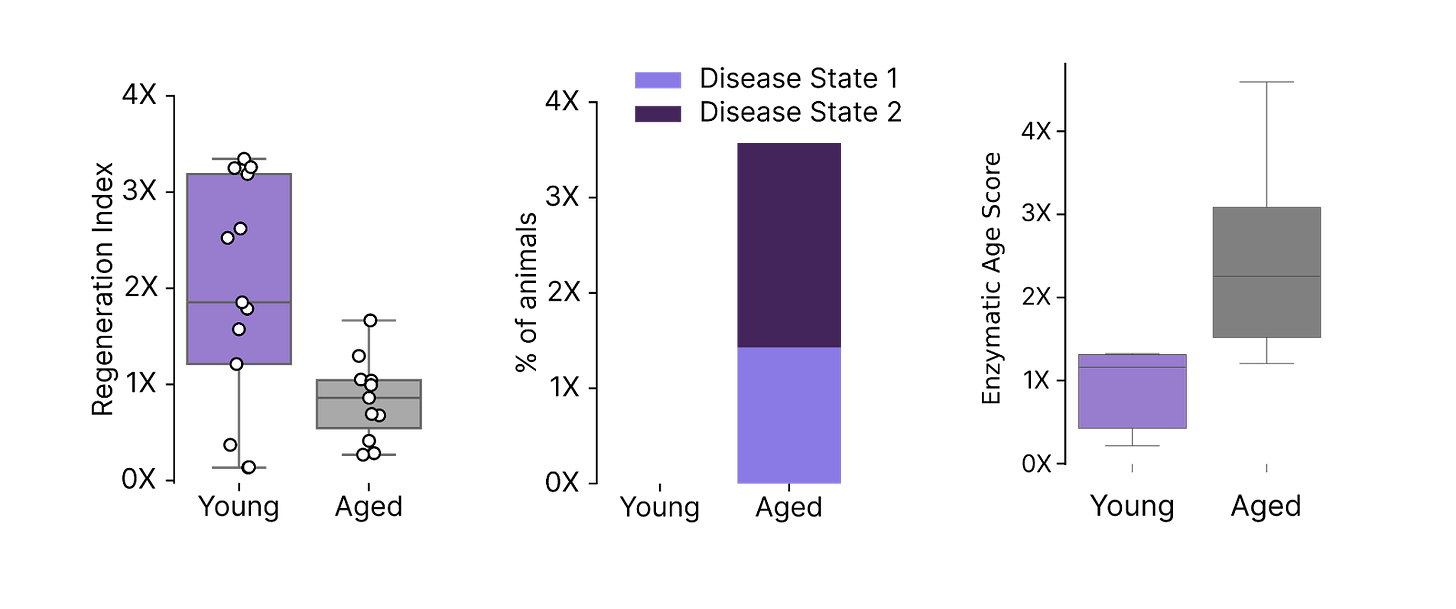
We’re looking for builders
We continue to expand our team across all levels of seniority. More so than any specific technical background, we’re excited to work with talented scientists, engineers, and operators who are passionate about our ambitious mission.
If you’re excited about our work, please reach out.