In the early months of the summer, we made geometric improvements to the screening tools in our Discovery Engine. Starting in July, we began using these tools to perform our largest screens to date.
As far as we know, these partial reprogramming screens are also the largest in the world to date. Promising early results from these experiments have triggered a rush of activity across our teams as we scale up for larger discovery campaigns.
More color on progress across our science & operations:
People
We’ve welcomed two new team members:
Shea Lambert is joining our Predict team as a Bioinformatics Engineer. Shea previously supported diverse products as a scientist at Inscripta.
Bryan McDonald is joining us as a Scientist on the Immunology team. Bryan began his career at Bristol Myers Squibb and trained with Susan Kaech at the Salk Institute where he explored epigenetic regulators of CD8 T cell differentiation.
Jenna Wolfley is joining our Operations team. Jenna was previously at GV (formerly Google Ventures) where she supported the life sciences investment team.
Atoms
Largest partial reprogramming screens to date
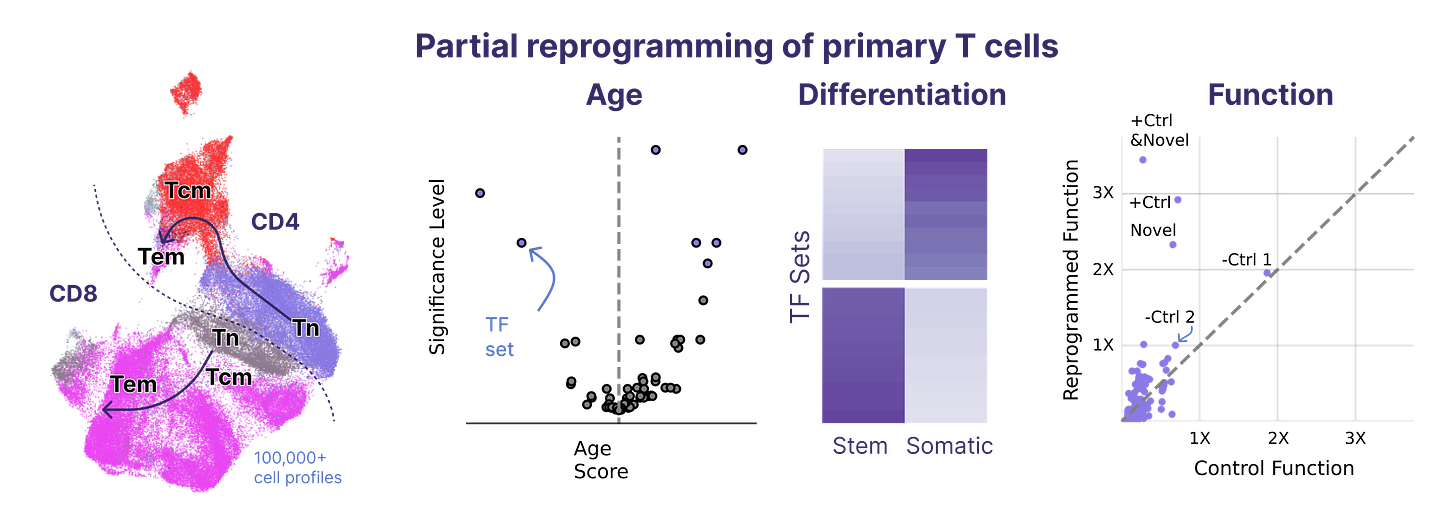
To our knowledge, we performed the world’s largest partial reprogramming screen to date in primary T cells over the past two months. We believe that the previous record was set by our screen in June.
We found that even within the limited pool of partial reprogramming factors we tested, we discovered TF sets sufficient to reprogram cell age, differentiation state, and cell function. This experiment served as the capstone on the development of our first generation screening technology.
There are still parts of our engine that rattle, pieces we’d like to polish and cover in chrome, and paint jobs that need to be applied. But we feel confident this is the first version of our technology suitable for scaling up. As a result, we’ve begun expanding our library of reprogramming factors to perform our screens oriented at therapeutic discovery, rather than technology development.
Reprogramming factor library manufacturing
Our Write team has been rapidly constructing that expanded reprogramming library. This represents the Build phase of our Discovery Engine’s Design-Build-Test-Learn cycle.
Six months ago, a single tick on the x-axis of the plot above took us multiple weeks to build. The same number of team members can now generate >2X the output in the same amount of time through a series of iterative process changes.
Expediting these Build times allows to us execute more cycles, increasing the overall learning rate of our organization.
Converting basepairs to bits under our own roof
While our labs in South San Francisco were being built out, we used external vendors to perform DNA sequencing. This allowed us to start up almost immediately, but we endured long turn around times as a result.
To reduce the time of the Test stages in our Discovery Engine, our Read and Operations teams brought DNA sequencing in-house. Their efforts have reduced our turn-around-time for sequencing by 5- to 10-fold, allowing us to take more shots on goal and learn more quickly.
Just as importantly, we now enjoy a lovely glow and hum in the lab as our libraries make their journey up to the cloud.
Bits
Harmonizing a reprogramming corpus
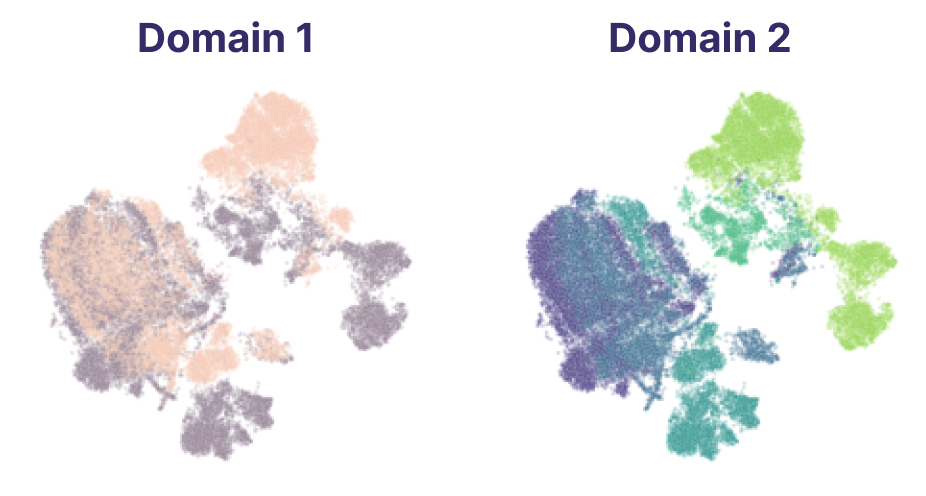
We’ve now generated a wealth of data to train our in silico reprogramming models, but the data from each individual screening experiment we’ve performed harbors slight differences. These experiment-specific differences are commonly known as batch effects in biology or domain shifts in machine learning.
In order to make the best use of our growing data compendium, this month we focused on developing tools to harmonize these experiments into a single, unified corpus. We’ve adopted a set of tools from machine learning to learn representations of cell state and reprogramming effects that are invariant to these experiment-specific nuances. Within these representations, we’ve found that we can reproduce partial reprogramming effects across experiments with high fidelity.
We can use these representations to compare reprogramming effects across factor combinations, even if those factors weren’t all tested at the same time. This harmonized data corpus serves as the substrate of reprogramming models, and we imagine the model’s capabilities will only increase as it grows.
Nominating reprogramming factors with deep models of cell identity & regulatory sequences
What reprogramming factors should we test, now that our screening chemistry is ready to scale? We primarily nominate factors using our in silico reprogramming models, but there are additional inductive biases that may prove fruitful as well. Another simple approach we’ve explored is simply asking: which factors change in activity with age?
We previously described an approach we’ve used nominate reprogramming factors based on an epigenetic map of T cell aging we built in January 2023. This month, we combined these data, our prior regulatory sequence model, and a new ML model that captures cell identity programs to nominate even more factors.
T cells contain a whole hierarchy of specialized subtypes that each age in a unique way. Using our learned models of cell identity, we were able to nominate reprogramming factors that might restore youthful function within specific T cell subsets. We look forward to learning how each of these heuristics performs in practice as we scale up our screens.