The opening months of the summer have been an energizing time at NewLimit. We’ve made geometric improvements on the performance of our reprogramming screening campaigns, built our first functional assays, and trained our first payload recommendation models using in-house data.
We also had the pleasure to welcome several new partners to the table with the close of our Series A.
As our Discovery Engine springs to life, we’re continuing to recruit talented builders across our Research and Operations departments. If you’d like to join us in developing reprogramming medicines, please reach out or apply on our website.
A few updates on our science & progress:
People
This month, we welcomed Ben Curtis as a Scientist on our Immunology team. Ben joins us from Michael Jensen’s lab at Seattle Children’s where he developed an innovative CRISPRa screening platform for primary T cells.
Atoms
High efficiency gene delivery in primary T cells
In April, we performed our first partial reprogramming screen in primary human T cells. This screen served as an exciting demonstration of our technology, but it’s only the v0 implementation of our Discovery Engine.
As with all v0 methods, there are many efficiency improvements to make!
Biochemical processes are traditionally evaluated along the dimensions of purity and yield. In short, purity is the fraction of your final output that actually contains your final product, and yield is the amount of your final output you can make. Usually, there’s a purity-yield trade-off at play as your optimize your process.
Our v0 T cell screening chemistry left room for improvement on both fronts. Given our approach, these issues largely stemmed from inefficient gene delivery.
In May and June, we iterated several times to implement a v1 of our chemistry that dramatically improved gene delivery performance and allowed us to increase purity by 2X and yield by >4X.
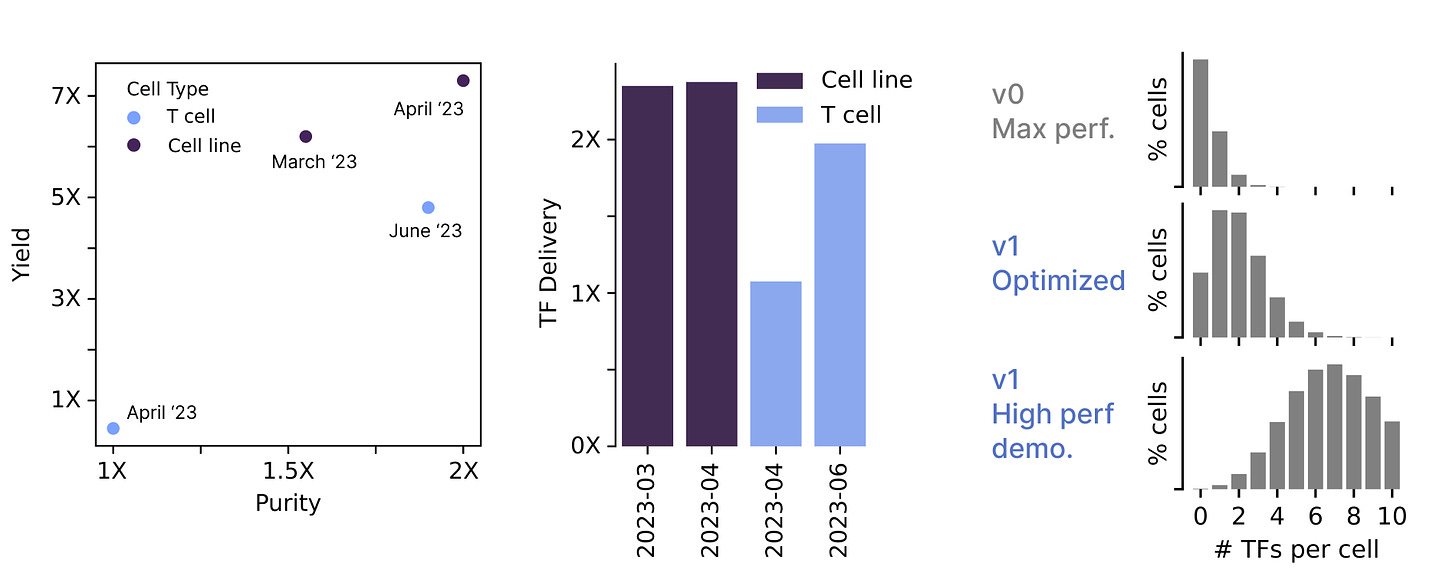
Function is the final boss
Our goal at NewLimit is to improve cell function to treat age-related diseases. Our Discovery Engine finds reprogramming factor sets that shift aged cell profiles to resemble youthful cell profiles, but this phenotypic similarity is only a hint that a reprogramming intervention might be therapeutic.
More than phenotypic similarity, we’re interested in testing if a reprogramming intervention improves cell function. In order to do that, we need a rigorous set of functional assays to evaluate the efficacy of reprogramming sets.
In June, our Immunology team developed the first three functional assays in our panel and found strong age-related differences across two of the three. With this bedrock in place, we can begin to validate early hits from our Discovery Engine.
Bits
Learned representations of cell age enable rejuvenation screens
In order to discover factors that reprogram cell age, we first need to be able to measure it.
Cell age is a tricky phenotype to quantify — there isn’t a singular molecular marker we can rely on to predict all of the functional consequences of aging that we’re interested in. Rather than searching for a single molecular biomarker, NewLimit is building machine learning models that allow us to infer cell age from cell profiles (previously discussed in our February 2023 Progress Update).
We’ve continued to expand and improve these models in the first half of 2023. Across three versions of our model, we’ve seen consistent performance gains as we accumulate a larger training set and improve our modeling approach.
In June we applied these methods for the first time to evaluate the impact of diverse reprogramming interventions on cell age in primary T cells.
This experiment is but the first in a series of many, but highlights how machine learning models enable to ask otherwise intractable biological questions like — “Does this reprogramming factor set make old cells look younger?”
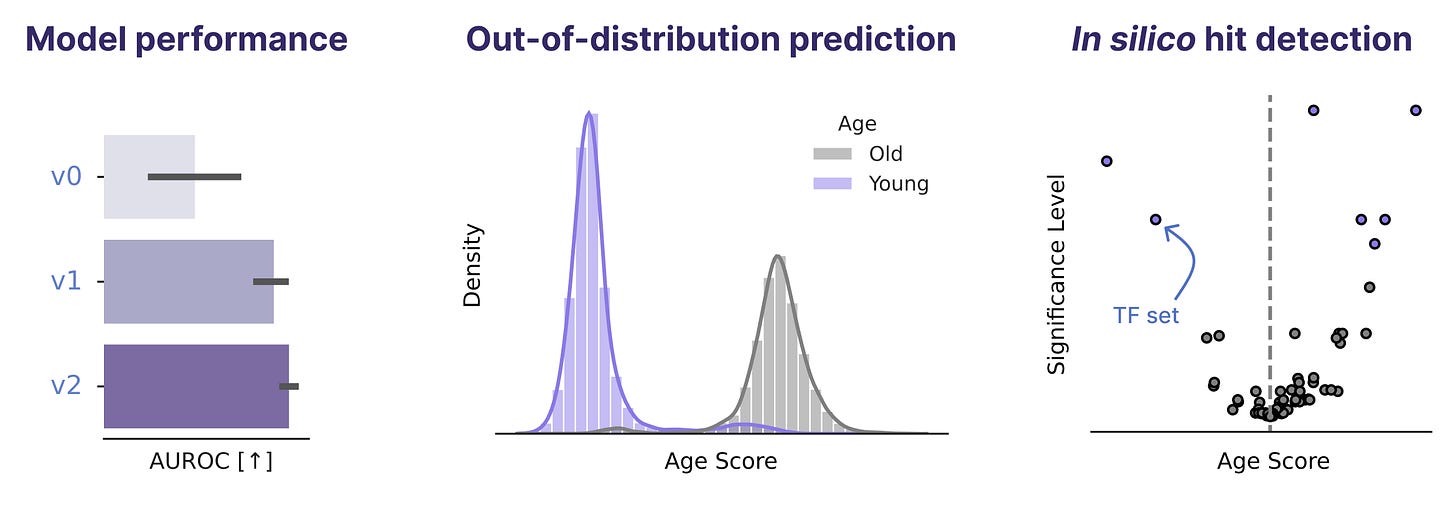
Recommending reprogramming payloads
No matter how clever we are in the world of atoms, we’ll never be able to test more than a small fraction of possible reprogramming sets.
We’re therefore building payload recommendation models to predict the effect of unseen reprogramming interventions and help select the most promising sets for our next round of experiments.
Our corpus of partial reprogramming data has grown large enough that we can now train our first in silico reprogramming models. Over the course of May and June, we’ve seen consistent performance improvements and now achieve performance superior to existing state-of-the-art methods.
This is still a very early version of our ultimate model, but we’re excited to see our modeling efforts yield dividends even early in the development of our Discovery Engine!